Part 6. Potential of atmospheric data to optimize model parameters
As an alternative of under-constrained "flux atmospheric inversions", recent data assimilation techniques proposed to optimize directly the parameters of ecosystem models using also the integrated information from atmospheric data, through the link of atmospheric transport models.
See main presentation for a description of the methodology.
As a first analysis, we investigate in this section the potential of current atmospheric network to optimise terrestrial model parameters, using a "pseudo-data" approach. The approach includes:
- The definition of pseudo atmospheric observations using a set of model parameters (TRUE parameters) to simulate surface fluxes (using ORCHIDEE model) that are further transported with an atmospheric transport model (LMDz)
- One or several parameters are randomly perturbated to produce a set of PRIOR parameters
- The optimisation system then tries to optimize the selected parameters (one or several) using a variational algorythm
- The optimized parameters are compared with the TRUE parameters
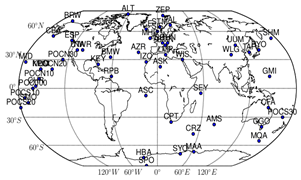
Figure 1. Atmospheric station location for this exercice.
Results with only one parameter:
Simple tests are presented in Table 1 with a 3 different parameters optimized independently. Each parameter is PFT-dependent (i.e. different value for different PFT). The meaning of the parameters can be found in Part 3.
Table. Result of different pseudo-obs assimilation for four different paramters: for Boreal needleleaf forest and tropical evergree forest
Parameters |
Q10 |
Vcmax_opt |
Fstressh |
Gsslope |
PFT |
|
Boreal |
Tropical |
Boreal |
Tropical |
Boreal |
Tropical |
Prior value |
2.502 |
77.672 |
28.6 |
5.4 |
7.6 |
6.744 |
10.2 |
Posterior value |
2.17 |
66.1148 |
35 |
7.7 |
5.99 |
7.2387 |
8.99 |
True value |
2.17 |
66.12 |
35 |
7.73 |
6 |
7.24 |
9 |
Results with more parameters:
Several test are presented in figure 2 with a given parameters but for different Plant functional type that are optimise simultaneously: from only one PFT to 9 PFTs. Note that the two parameters considered here are PFT dependant in ORCHIDEE so that the case with N PFTs correspond to an optimisation with N parameters (all independent).
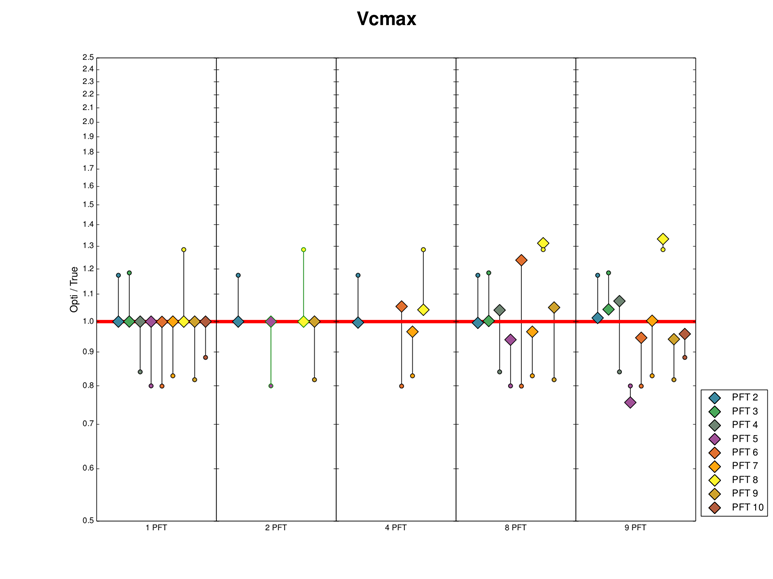
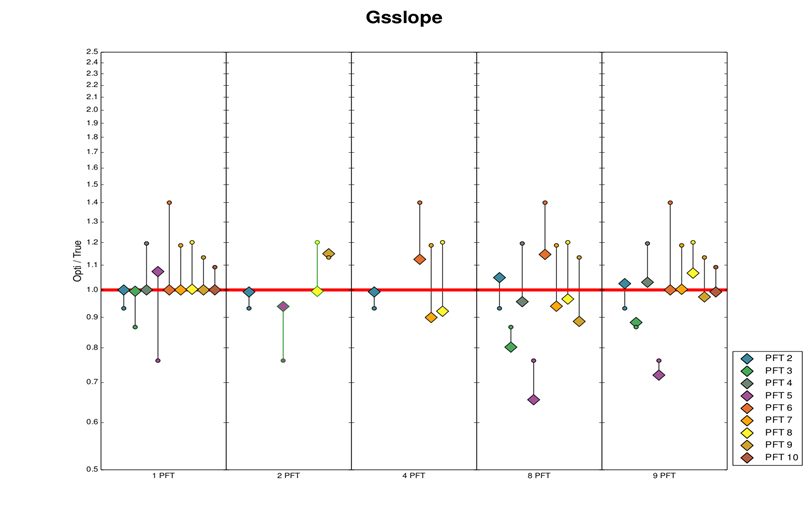
Figure 2. Pseudo-obs assimilation for two different parameters : Vcmax_opt (top), and Gsslope (bottom). For each parameter, pseudo-obs assimilation have been processes for only one PFT (first column), two PFTs (second column), four PFTs (third column), eight PFTs (fourth column) and nine PFTs (fifth column). For two PFTs, two couples of PFTs have been chosen and the lines appear in different color for each. The red line shows the true value of the parameter. The small symbols represent the PRIOR parameter value and the big symbol represents the POSTERIOR value.
Questions:
- From the results of table 1, what do you conclude?
- From the results of figure 2, what do we learn on the potential of atmospheric CO2 data? Why do you think the posterior values do not converge to the TRUE values when having many PFTs (equivalent to many parameters)?