Part 5. Assimilation of fluxnet data: single vs multiple sites
In a separate study we have used the variational (gradient-descent) method to optimize different PFTs of the ORCHIDEE model using FluxNet data (Figure 1). The results shown here are for the optimization of the temperate broadleaved deciduous forest PFT in ORCHIDEE using data from 12 sites across the globe (Table 1). These results are described in Kuppel et al., (2012). In a further study (Kuppel et al., 2014, submitted), between 2 and 24 sites have been assimilated per PFT from stations across the globe depending on the data availability.
Both NEE and LE daily fluxes were assimilated both at each site (single-site — SS) and for all sites for the same PFT (multi-site — MS — optimization). Many studies have used FluxNet data to optimize ecosystem/land surface models in the past, but none have used all the sites together in the same optimization for such a range of PFTs.
A MS optimization is important, because for global simulations we need to have one parameter vector per PFT. If we just optimize at each site, we may find a range of posterior parameter values for each parameter for the same PFT. In order to do global simulations therefore, should the average be taken? Or is it better to include all sites in the same optimization? The aim of this study is therefore to see if the MS optimization performs as well as the SS optimization and/or the average of the SS optimisations.
The number of years assimilated is different for each site (see Table 1). The parameters that were optimized are described in Table 2. It was assumed that there was no correlations between parameters. The errors on the observations were given as the prior RMSE between the model and the observation, and were assumed to be independent (i.e. no correlation).
Table 1. Sites used in this study, with their name code made from the country (first two letters) and site name (last three letters) (Kuppel et al., 2014)
Site |
Location |
Main tree species |
Stand age |
LAI |
Period |
References |
DE-Hai |
51.079° N, 10.452° E |
Beech, ash, maple |
1-250 |
5 |
2000-2006 |
Knohl et al. (2003) |
DK-Sor |
55.487° N, 11.646° E |
European beech |
84 |
4.8 |
2004-2006 |
Pilegaard et al. (2001) |
FR-Fon |
48.476° N, 2.78° E |
Oak |
100-150 |
5.1 |
2006 |
Prevost-Boure et al. (2010) |
FR-Hes |
48.674° N, 7.064° E |
European beech |
35 |
4.8-7.6 |
2001-2003 |
Granier et al. (2008) |
JP-Tak |
36.146° N, 137.423° E |
Oak, birch |
50 |
— |
1999-2004 |
Ito et al. (2006) |
UK-Ham |
51.121° N, 0.861° W |
Oak |
70 |
— |
2004-2005 |
http://www.forestry.gov.uk |
US-Bar |
44.065° N, 71.288° W |
American beech, sugar, yellow birch |
73 |
3.6-4.5 |
2004-2005 |
Jenkins et al. (2007) |
US-Hal |
42.538° N, 72.172° W |
Red oak, red maple |
75-110 |
— |
2003-2006 |
Urbanski et al. (2007) |
US-LPH |
42.542° N, 72.185° W |
Red oak |
45-110 |
4-5 |
2003-2004 |
Hadley et al. (2008) |
US-MOz |
38.744° N, 92.2° W |
White & black oaks, shagbark hickory, sugar maple, eastern red cedar |
— |
— |
2005-2006 |
Gu et al. (2012) |
US-UMB |
45.56° N, 84.714° W |
Bigtooth aspen, trembling aspen |
90 |
3.7 |
1999-2003 |
Curtis et al. (2002) |
US-WCr |
45.806° N, 90.08° W |
Sugar maple, basswood, green ash |
60-80 |
5.3 |
1999-2004 |
Cook et al. (2004) |
Table 2. ORCHIDEE parameters optimized in this study (Kuppel et al., 2012)
Parameter |
Description |
Prior value |
Prior range |
σprior |
Photosynthesis |
Vcmax |
Maximum carboxylation rate (μmol m–2s–1) |
55 |
27-110 |
33.2 |
Gs,slope |
Ball-Berry slope |
9 |
3-15 |
4.8 |
Topt |
Optimal photosynthesis temperature (°C) |
26 |
6-46 |
16 |
Tmin |
Minimal photosynthesis temperature (°C) |
–2 |
(–7)–3 |
4 |
SLA |
Specific leaf area (LAI per dry matter content, m2g–1) |
0.026 |
0.013-0.05 |
0.0148 |
LAImax |
Maximum LAI per PFT (m2m–2) |
5 |
3-7 |
1.6 |
Klai,happy |
LAI threshold to stop carbohydrate use |
0.5 |
0.35-0.7 |
0.14 |
Phenology |
Kpheno,crit |
Multiplicative factor for growing season start threshold |
1 |
0.5-2 |
0.6 |
Tsenses |
Temperature threshold for senescence (°C) |
12 |
2-22 |
8 |
Lagecrit |
Average critical age for leaves (days) |
180 |
80-280 |
80 |
Soil water availability |
Humcste |
Root profile (m–1) |
0.8 |
0.2-3 |
1.12 |
Dpucste |
Total depth of soil water pool (m) |
2 |
0.1-6 |
2.36 |
Respiration |
Q10 |
Temperature dependence of heterotrophic respiration |
1.99372 |
1-3 |
0.8 |
KsoilC |
Multiplicative factor of initial carbon pools |
1 |
0.1-2 |
0.76 |
HRH,b |
First-degree coefficient of the function for moisture control factor of heterotrophic respiration |
2.4 |
2.1-2.7 |
0.24 |
HRH,c |
Offset of the function for moisture control factor of heterotrophic respiration |
–0.29 |
(–0.59)–0.01 |
0.24 |
MRa |
Slope of the affine relationship between temperature and maintenance respiration |
0.16 |
0.08-0.24 |
0.064 |
MRb |
Offset of the affine relationship between temperature and maintenance respiration |
1 |
0.1-2 |
0.76 |
GRfrac |
Fraction of biomass available for growth respiration |
0.28 |
0.2-0.36 |
0.064 |
Energy balance |
Z0overheight |
Characteristic rugosity length (m) |
0.0625 |
0.02-0.1 |
0.032 |
Kalbedo,veg |
Multiplying factor for surface albedo |
1 |
0.8-1.2 |
0.16 |
SECTION A
Examine the time series below from two temperate broadleaved deciduous forest (TempDBF) sites in France and the US when optimizing with NEE and LE data and answer the following questions.
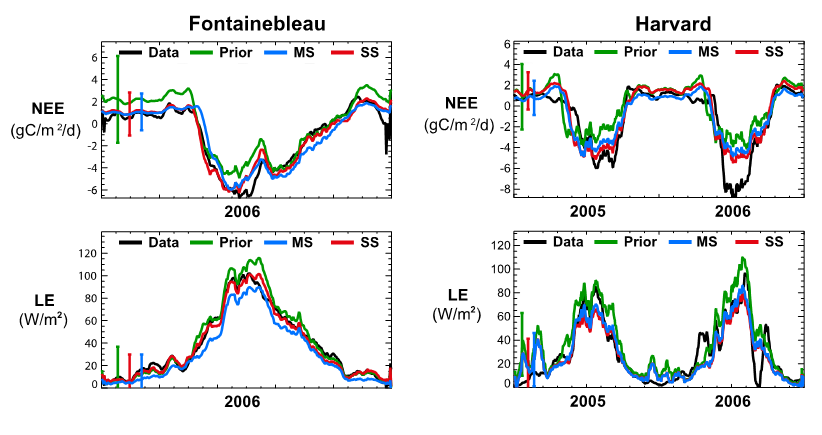
Figure. Caption
- Does the optimization result in a better fit to the observations in all cases?
- Does the multi-site optimization work as well as the single-site? What are the reasons for the differences?
- What do you think is causing any remaining discrepancies between the model and data after optimisation?
SECTION B
Now take a look at the following figure:
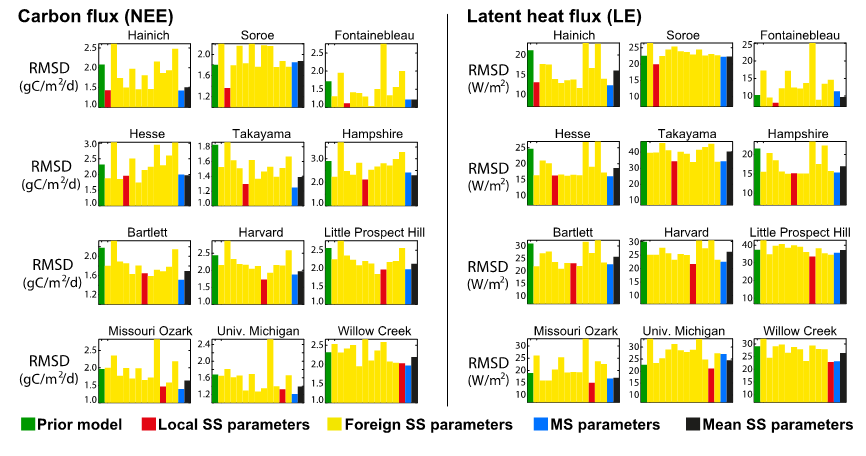
Figure. Caption
Compare the RMSD using the parameters derived from the optimization at each site (Local SS parameters) to the RMSD from parameters derived in the MS optimization (MS parameters), and to the parameters derived from single-site optimization from other sites (Foreign SS parameters) and to the mean of the SS parameters. Answer the questions below:
- If you use parameters derived from a single site optimisation at another site (yellow), does it result in a similar RMSD between the model and observations?
- Why is there such a variety in the model-observation RMSD when parameters derived from single-site optimisations at other sites are used? What are the differences between sites?
- Comment on the MS (blue) performances versus the SS (red) optimization at each site. What could be the cause of a better fit to the data with the MS optimisation than with the SS optimization?
- What are the differences between the mean of all the single-site optimisations versus the simulations using parameters derived in the MS optimisation? Which do you think would be better for global simulations?
SECTION C
Now take a look at the posterior parameter values in the figure below (grey = prior, black = MS value, colours = each SS optimization):
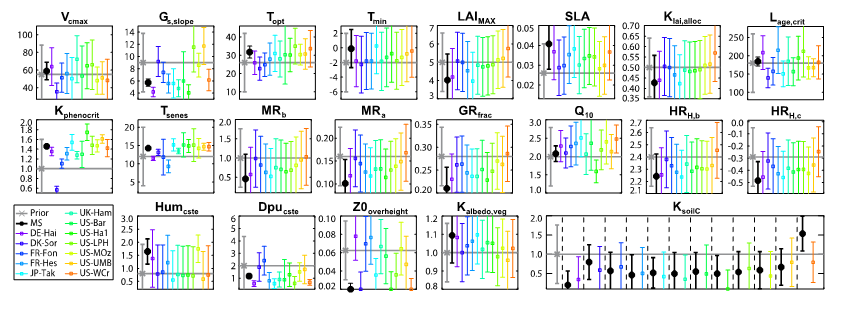
Figure. Caption
SECTION A
- Does the optimization result in a better fit to the observations in all cases?
- Does the multi-site optimization work as well as the single-site? What are the reasons for the differences?
- What do you think is causing any remaining discrepancies between the model and data after optimisation?
SECTION B
- If you use parameters derived from a single site optimisation at another site (yellow), does it result in a similar RMSD between the model and observations?
- Why is there such a variety in the model-observation RMSD when parameters derived from single-site optimisations at other sites are used? What are the differences between sites?
- Comment on the MS (blue) performances versus the SS (red) optimization at each site. What could be the cause of a better fit to the data with the MS optimisation than with the SS optimization?
- What are the differences between the mean of all the single-site optimisations versus the simulations using parameters derived in the MS optimisation? Which do you think would be better for global simulations?
SECTION C
- Which processes do we constrain the most (refer to Table 2)?
- Why do you think there is such variation for some parameters between the sites?
- Why do you think the MS parameter values do not approximate the mean of the SS parameters?